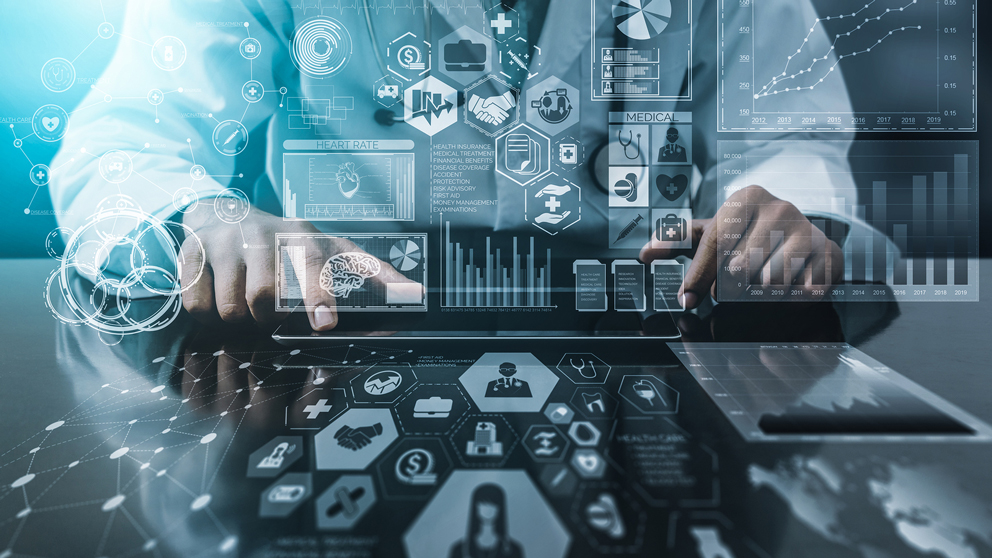
There has been an explosion of digital medical data in recent years, taking many forms. Much of the most valuable data—clinical patient data—is currently stored in electronic health record (EHR) systems, providing a theoretical gold mine for large-scale integration and analyses of patient traits, diseases, treatments, progression over time, outcomes and more.
Unfortunately, EHRs have limited interoperability, hindering access to data across healthcare systems. There is also poor standardization for the data itself, meaning that the same traits, treatments, outcomes, etc. are represented in multiple ways within the records themselves.
In a recent paper published in the New England Journal of Medicine, Jackson Laboratory (JAX) Professor Peter Robinson, M.D., M.SDevelops algorithms and software for the analysis of exome and genome sequences. Peter Robinson, M.D., M.Sc. and co-authors had assessed the problems involved and the barriers to solving them. To overcome many of the barriers and truly implement data-driven precision medicine, they presented their case for applying ontologies, specialized computational representations of specific subject areas such as medicine, that provide logical consistency across large numbers of terms and concepts. Now, in a paper appearing in npj Digital Medicine, Robinson and Xingmin Aaron Zhang, Ph.D.Patient deep phenotyping from various data types in electronic health records; phenotype- and genomic-based disease modeling and algorithm development for translational research and differential diagnosesPostdoctoral Associate Aaron Zhang, Ph.D., led a large team that showed how converting EHR entries to ontology-based terms allows researchers to gain far more insight from patient data.
The power and potential of patient data
For the study, Robinson, Zhang and colleagues focused on laboratory test results, which in themselves contain a significant amount of patient trait and clinical information. Tests performed for the same symptoms can vary, however, and the results can be presented in various formats (e.g., a specific number or high/normal/low). Nonetheless, a universal code system known as the Laboratory Observation Identifier Names and Codes (LOINC) has been adopted within most EHRs to define laboratory tests. Using the Fast Healthcare Interoperability resource, an interoperability tool that provides a standardized interface to EHR systems, the researchers were able to develop an algorithm to map LOINC-encoded test results to terms implemented in the Human Phenotype Ontology, the current standard for computational trait analysis. The process automatically extracts detailed, deep phenotypic profiles of laboratory test results for subsequent analysis.
Just what can be done with such a process? To provide a working example, the team implemented their method using a de-identified EHR dataset comprising more than 15,000 patients with histories of asthma or asthma-like symptoms. The dataset contained a staggering 54 million records, with 11 million clinical test results, plus prescriptions, diagnostic codes and other patient information and records. Using the algorithm, the researchers successfully migrated more than 88% of the test results to Human Phenotype Ontology terms, which they then used to identify abnormalities associated with an asthma diagnosis or with frequent medication (prednisone) use. For example, the ontology analysis yielded connections with seven traits that co-occur with a diagnosis of severe asthma. Importantly, some of the conditions identified, such as abnormal vitamin metabolism and abnormal eosinophil (a form of immune cell) count, have previously been associated with various aspects of asthma but only through expensive and time-consuming clinical studies.
The insights gained through use of the ontology can help advance clinical progress, such as through the development of diagnostic tools. Some barriers still exist, as the method depends on implementation of LOINC and the interoperability tool, and it’s not universally applicable at this time. Nonetheless, the authors anticipate that the ability to integrate EHR data with a working ontology will expand over time. Moving forward, the data will be leveraged to support data-driven translational research and accelerate the implementation of precision medicine.