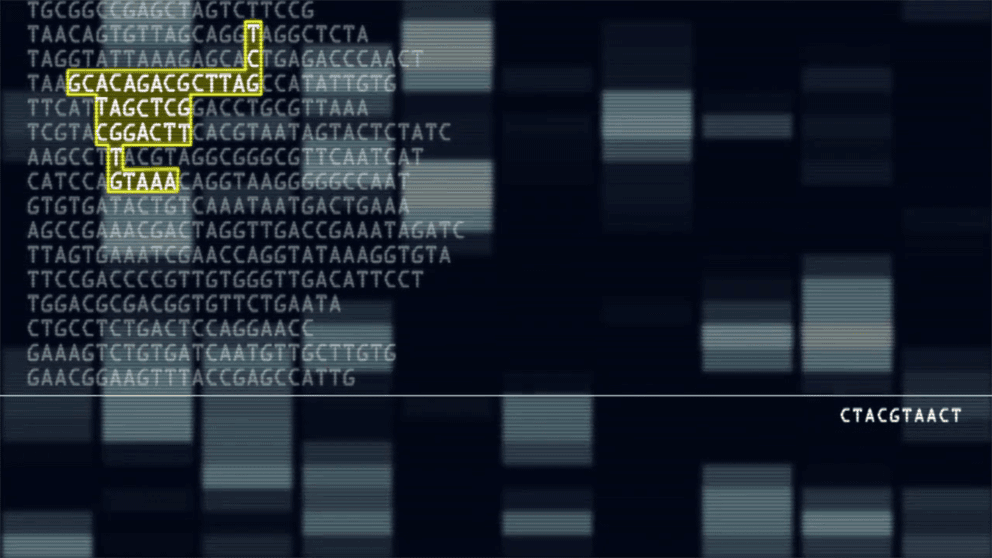
The advent of precision medicine, also known as personalized medicine, has been predicted and discussed in earnest since the publication of the first human genome sequence in 2003. Its cornerstone concept is that we can learn far more about the health of each of us by embracing all of our data, not focusing only on the relatively small amount that can be obtained from visits to the clinic. We can now obtain those data points relatively easily, but difficulties with managing them in ways that can actually improve medicine — sharing them, integrating them across patient populations and data types, analyzing them, and so on — remain a significant barrier to progress.
In a paper published in New England Journal of Medicine, “Enabling Precision Medicine — Classification, Ontology, and Computational Reasoning,” JAX Professor Peter Robinson and colleagues propose how to adjust the medical data infrastructure to overcome the current obstacles.
Exactly what data are being obtained? Think about a typical doctor’s office visit. There are the easy ones: height, weight, blood pressure, pulse, temperature. With a blood draw add cholesterol, blood sugar, and any number of other numbers, if requested, such as thyroid function measures, PSA level (for males), vitamin levels, and so on. A short Q & A assesses history, current behavior and environment, e.g., smoking, exercise, and dietary habits, medications taken, pets in the house, family history of disease, and so on. For a long time, that was about all there was.
Nowadays, you can add genome or exome sequences; RNA sequencing (which genes are active or not); proteomics (the actual proteins present, and at what levels); CAT scans, MRI and/or other imaging; and other measurements and test results that essentially reveal a patient’s biology. There’s also patient reported data, including the emerging trend of wearable data and other real-time inputs generated outside of the clinic, sometimes on a 24/7 basis.
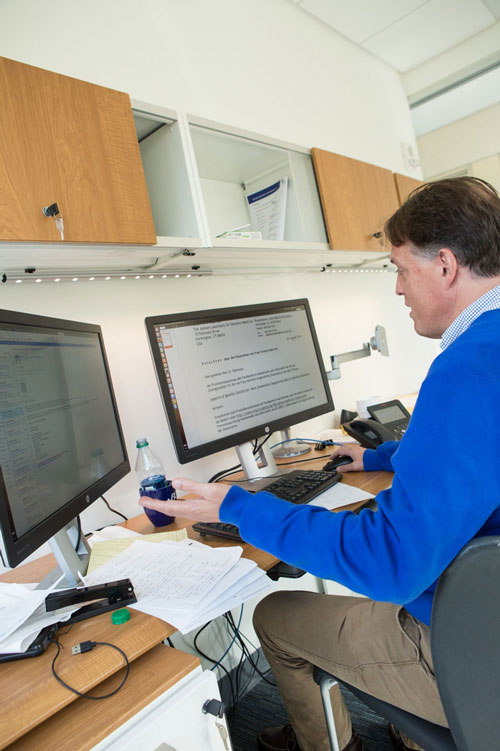
For a doctor practicing in the current environment, all of these data can pose a significant challenge. Modern electronic health records (EHRs) have been designed more with billing purposes in mind than making these new patient data standardized and interoperable. And current medical classification and data semantics schema have limited portability to computing environments and do not even correspond with one another at times.
A notable example is the International Classification of Diseases, first published in 1891 and now on its 10th iteration. ICD10 limits each code to one and only one “parent” to which it is linked. As Robinson notes, this means that “Malignant neoplasm [cancer] of thyroid gland” is a child of “Malignant neoplasms” but not also “Disorders of thyroid gland,” severely limiting its use in making connections between patients, diseases, and populations. (Note: this also makes for some absurdly specific ICD10 codes, given the hyper-granularity required. Hopefully little-used codes include V91.07 (Burn due to water skis on fire) and T63.012A (Toxic effect of rattlesnake venom, intentional self-harm)).
So what solutions are available?
In the paper, the authors argue that there needs to be a concerted effort “to align data across patients and systems with comparable and consistent formats and contextual meaning.”
A way to do this is to implement ontologies in medicine. Ontologies are essentially sets of terms about a specific subject that not only describe the properties of the terms but also specify the relationships between them. In modern usage, ontologies are often thought of as a computational representation of a specific subject area, such as medicine. Correctly implemented, an ontology can provide logical consistency across huge numbers of terms and concepts. In medicine they can help transcend EHR limitations by integrating basic science data with clinical data to improve patient classification as well as diagnostic and therapeutic insight.
Ontologies are already helping to advance the genetic diagnosis of rare diseases in translational research settings. Finding clinical meaning in ~4.5 million genomic variants combined with phenotypic (trait) abnormalities is difficult, but computational analysis systems that use ontologies contextualize the sequence data within the assessment of patient traits. The efforts include the Human Phenotype Ontology (HPO), an approach that is designed for computational analysis by linking disease definitions with ontologies on gene function, anatomy, biochemistry, and other biological attributes. Basically, the HPO represents the patient as a biological subject, not a bill-payer, enabling far more powerful individual diagnostic analyses as well as the identification of patients with similar disease phenotypes. Robinson initiated the HPO in 2008 and continues to lead its development.
In the end, Robinson envisions a medical ecosystem in which a doctor can ask and/or answer a variety of questions: “Which axes of patient characteristics bear scrutiny? Demographics, signs, symptoms, family history, diagnoses, anthropometrics, test results, radiology, or “omics” measures? How much of these data are already in my patient’s record? How much are in the records of putative patients like mine? How big is the universe of corresponding data that I can examine, in my practice, in my hospital, in my group, in my state, in my country?”
In addition to the task of implementing ontologies, he identifies three primary barriers to realizing the vision, however. Figuring out patient privacy and security issues in the context of laws enacted before access to medical data became so valuable is one. The EHR issue, including lack of interoperability, proprietary interfaces, and non-standard data structures, is another. Finally, integration of additional data sources with EHRs and each other, including public research databases and clinical references, is needed to break down data siloes and maximize data value across larger patient populations.
Overcoming these barriers within an entrenched system can be a daunting process, but the time is right, and the potential benefits are enormous. Aggregating and integrating each individual’s data will provide a depiction of the patient as an entire system, with embedded interrelationships. Combining such systems-level data collections with those of millions of other patients will reveal population-level patterns and how individuals vary from them.
We are on the threshold of insights that will transform the field of medicine. Once barriers are removed and effective data management is enabled, we will realize them.