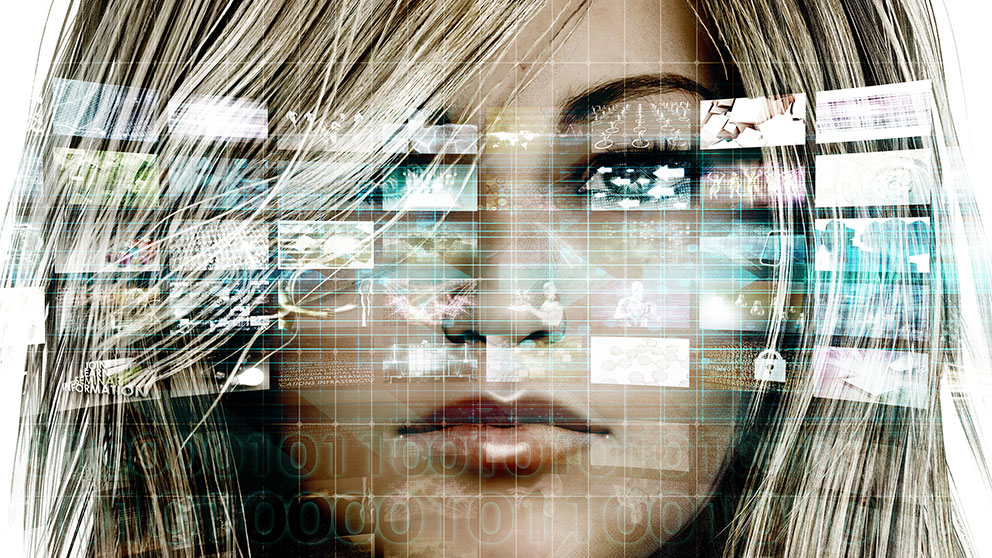
The term "big data" is a buzz phrase that can be misleading. So what is big data supposed to mean? And why is big data so important in our daily lives?
At its core, big data is just that — a huge amount of information, sometimes from a huge number of sources. Analyzing it takes special equipment and special skill sets. Imagine an Excel file, for example. Any worksheet with more than a few hundred lines makes it unwieldy for all but the most skillful users. Now imagine a worksheet with billions, maybe trillions of lines. Right. That’s why it takes embracing the complexity of massive data sets rather than seeking to reduce them to a manageable scope. Otherwise, big data provides no more insight than just, well, data.
In industry, big data is already being used in myriad ways, and all of us generate countless data points every day whether we like it or not. From suggesting the next song for a mix to making sure a package arrives on time (and knowing exactly what’s in it) to predicting which shoes we’ll buy next, our tastes and habits are constantly tracked, analyzed, modeled, and served back to us in various forms.
In medicine, the data points have been relatively few until recently. Outside of the clinic they pretty much haven’t existed, though wearables are beginning to provide enough real-time data for researchers to tap. For healthy people, a yearly physical yields some data — baseline blood pressure, cholesterol, weight, prescriptions, etc. — though it’s not exactly “big.” But with the advent of human genome sequencing and other large-scale molecular assays, researchers and clinicians can now examine certain populations in ways that do, in fact, present data challenges.
Each human genome has roughly 3.2 billion base pairs, roughly 20,000 genes, and more than 10 million SNPs (natural variants between individual genomes). They also have huge numbers of regulatory regions that may not code for genes but do affect if genes are expressed and at what levels. So genome sequences alone constitute big data, especially when grouped by the thousands and tens of thousands.
But there’s much more. Every genome has epigenetic markers, chemical groups attached to the DNA that affect gene expression. Each cell has what’s called the transcriptome, which is all the RNA coded for by the DNA. And the proteome, predictably, is comprised of all the proteins present at a given time. Then there’s phenotype, the basics of which are the BP, weight, cholesterol, etc. obtained from the physical. But in-depth phenotyping contains countless more measurements, including metabolism, activity level, physical state (including internal imaging such as MRIs) within the same tissues, in different tissues (where they will likely yield different data), and on and on.
All the data — billions and billions of data points in different formats and measuring different things — adds up to define the state of an organism. In the clinic, it’s a human, in research it’s often a mouse. What we’ve learned over the years is that for complex diseases that involve many different genes and systems as well as behavior and environment, a big data approach that embraces this complexity is needed to see what’s really happening in patients, and why. It’s also needed to bring the patient insight to an experimental setting, such as one that uses mice, to learn more about its initiation, pathology and component pieces. Finally, and most importantly, it’s needed to develop and test compounds that might block disease progression or prevent it from occurring in the first place.
So the next time you look at a spreadsheet in dismay as the rows disappear off the bottom of the window, think about working with several billion more rows and combining worksheets that have different headers and different field formats in every column. You’ll then have an inkling of what faces computational biologists working to overcome medicine’s most-difficult-to-cure diseases.