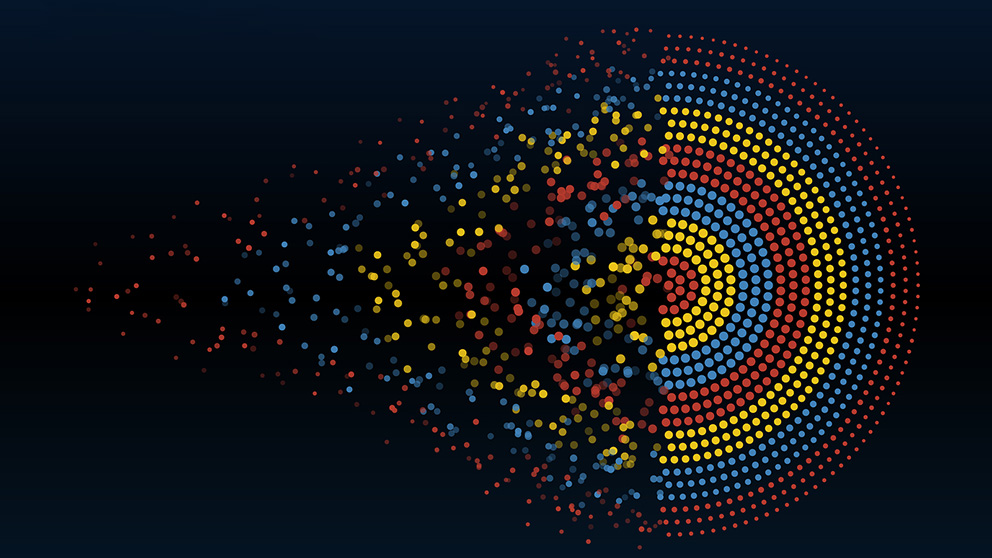
Structural variants, or SVs, are large DNA sequences that are inserted, inverted, deleted or duplicated within genomes. Finding SVs with short-read seq and analysis methods is difficult, but a new SV identification tool, FusorSV, sets a gold standard for SV detection and analysis.
In theory, our genomes are tidy, if huge. Most of us have two copies of each of 22 chromosomes, plus XX or XY sex chromosome combinations. Nucleotide sequences differ between people here and there, but for the most part, we share very similar genomes. In truth, however, things are somewhat messier.
Structural variants (SVs) in the genome — where segments of DNA are duplicated, deleted, inverted, inserted, translocated and more — are far more common than originally expected, even in outwardly healthy people. Indeed, on average they provide about five times more genetic variability between individuals than single nucleotide differences. And as we learn more about genomes, we’re finding out that SVs can also be important contributors to disease, including many cancers.
One reason knowledge and understanding about SVs have been slow to accumulate is that they are very difficult to identify in high throughput sequencing, especially short-read sequencing, the most common method. It makes sense — when the genome is cut up into small pieces and then reassembled it’s relatively easy to construct a linear sequence. But it’s difficult to detect when the sequence is repeated more than twice, or fewer, or is backward in places, or is in a different place, and so on. The assemblers will often “fix” the sequences by aligning them with the reference sequence, obscuring the SV. So even though single nucleotide variants can be called with high accuracy, existing SV algorithms lack that power.
To address the problem, researchers led by JAX Research Scientist , and Professor Charles Lee, Ph.D., FACMGThe study of structural genomic variation in human biology, evolution and disease.Charles Lee, Ph.D. , constructed a new SV identification tool, FusorSV, that combines eight existing calling methods with a new algorithm, built using 27 genomes that have undergone deep coverage from the 1000 Genome Project. In a paper published in Genome Biology, the researchers present their data mining approach to assessing algorithm performance and merging the output from the existing calling tools. The result is a more sensitive SV caller with increased accuracy.
Using FusorSV, the team identified 843 novel SV calls (610 deletions, 202 duplications and 31 inversions) that had not been previously reported in the 27 genomes. Going further, a subset of the calls were validated at an overall rate of 86.7%. The authors are sharing FusorSV with the research community in hopes that it will serve as the new gold standard for SV calling.
Becker et al. FusorSV: an algorithm for optimally combining data from multiple structural variation detection methods. Genome Biology (2018) 19:38 doi.org/10.1186/s13059-018-1404-6