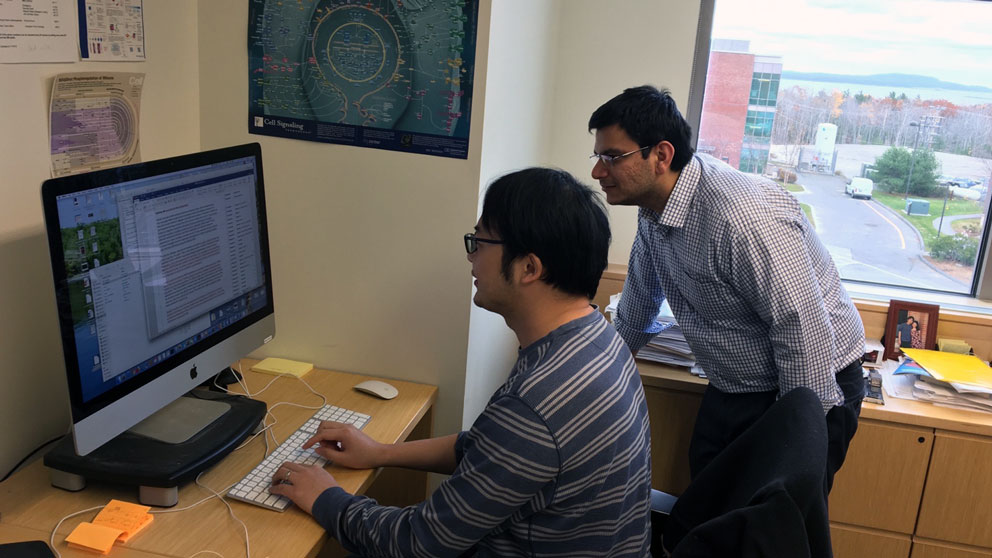
JAX researchers have developed a new computational algorithm that is capable of modeling the effects of both stochastic gene expression and cell-to-cell variability in a cell population.
Cellular processes involve myriad simultaneous molecular actions. Genes are expressed (or not); messenger RNAs are spliced then translated to proteins then degrade; proteins act or have their function blocked or degrade or bind with other proteins or molecules or receptors or are transported (perhaps outside of the cell or into the nucleus); and on and on. Thus, the vast networks of regulatory checkpoints and cellular functions comprising the cell involve uncertainty resulting in variability among the cells in a population. Measuring all of these molecular machinations directly in real time is impossible, and traditional biology was reductionist as a result, focusing on one piece at time. To truly understand function, however, it’s vital to investigate how the components work together in a large cell population despite these stochastic (random) differences among the individual cells.
To meet this challenge, computational methods are needed to provide a systems-biology view of gene regulation. In a paper, “Role of noise and parametric variation in the dynamics of gene regulatory circuits” published in npj Systems Biology and Applications, Assistant Professor Mingyang Lu, Ph.D. and Research Scientist Vivek Kohar, Ph.D. developed a new computational algorithm, sRACIPE (stochastic random circuit perturbation) to model the dynamic behavior of gene regulatory circuits. The method is capable of modeling the effects of both the stochastic gene expression and cell-to-cell variability in a cell population.
Unlike conventional methods, sRACIPE generates an ensemble of possible models for a holistic view of the circuit dynamics, therefore uniquely converting a traditional nonlinear dynamics problem into a data analysis problem. This feature allows researchers to study large complex populations. They tested sRACIPE on synthetic gene regulatory circuits as well as a well-studied epithelial-mesenchymal transition network in squamous cell carcinoma and demonstrated that the simulation results from sRACIPE were closer to experimental observations from single cell expression data than conventional methods. The authors have made the sRACIPE package freely available for academic use.
Research reported in this study was supported by the National Institute of General Medical Sciences of the National Institutes of Health under Award Number R35GM128717. The content is solely the responsibility of the authors and does not necessarily represent the official views of the National Institutes of Health.