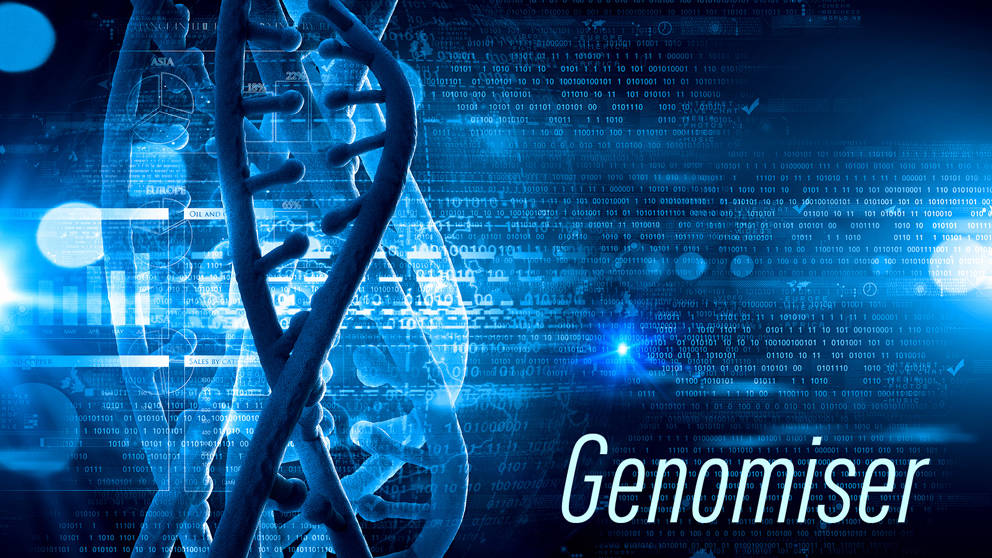
Exome sequencing (WES), which focuses on only the approximately 1.5% of the genome that codes for proteins, has proven to be effective in diagnosing rare Mendelian diseases. Multiple programs report a success rate of around 30% for diagnosing previously undiagnosed patients. At the same time, whole genome sequencing (WGS) is becoming increasingly feasible and relevant in medical genetics and can detect a broader range of genetic variations than WES, including structural variants such as copy-number variants and translocations. It also covers the full genome, including non-coding regions that have been found to play key roles in gene regulation and some human diseases.
Indeed, most of the genetic variants found associated with human disease were found in non-coding regions, but they remain poorly understood. As a result, Mendelian disease analysis retains an observational bias toward exome sequences, and regulatory mutations in non-coding regions are rarely reported. So while a 30% success rate for WES in clinical settings is laudable, it’s clear that medical genetics needs better tools to detect regulatory variants that underlie Mendelian disease in many other patients.
To accelerate the process, researchers led by JAX Professor Peter Robinson, Ph.D., developed “Genomiser,” a new tool that combines machine learning and an algorithm for ranking non-coding variants. Presented in paper published last week in The American Journal of Human Genetics, Genomiser represents a step forward in two areas. First, recently developed machine learning tools now detect single nucleotide variants related to disease or genetic regulation in coding regions, but none previously existed for non-coding variants. Also, its algorithm factors in phenotypes, coding region variants, non-coding variants and existing gene-phenotype associations. Inclusion of phenotype data distinguishes Genomiser from other methods, which are designed to assess the potential deleteriousness/pathogenicity of a gene variant irrespective of disease.
When running 10,000 simulations on diagnostic genomes, Genomiser identified the correct regulatory variant as the first choice in 77% of the cases. While a 77% success rate won’t happen in the real world where the diagnostic variant is unknown — the simulation genomes all had a diagnostic solution in the non-coding sequence — Genomiser will greatly improve clinical the diagnostic power of WGS data by accurately analyzing non-coding variants. It is is freely available as a download and will process a whole genome in around 10 minutes on a standard desktop computer. As the authors conclude: “This approach has the potential to substantially accelerate the detection of pathogenic, non-coding Mendelian variants by NGS and to explore the role of this currently understudied category of mutations.”
Smedley et al., A Whole-Genome Analysis Framework for Effective Identification of Pathogenic Regulatory. Variants in Mendelian Disease, The American Journal of Human Genetics (2016), http://dx.doi.org/10.1016/j.ajhg.2016.07.005